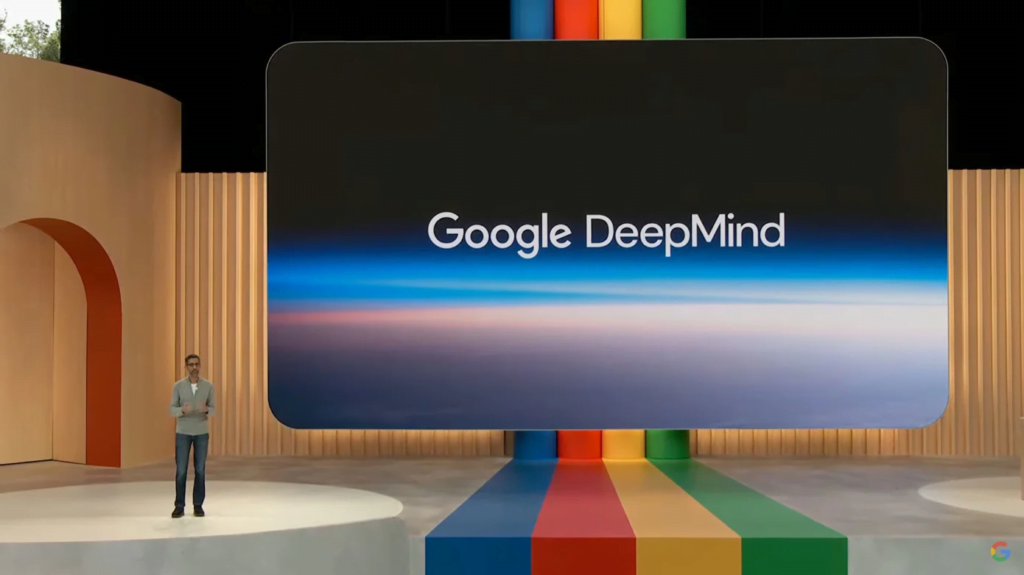
(Image credit: Google)
DeepMind, the renowned artificial intelligence (AI) research lab, has unveiled its groundbreaking creation, RoboCat. This state-of-the-art AI model is designed to perform a diverse range of tasks across various models of robotic arms. What sets RoboCat apart is its exceptional ability to not only solve multiple tasks but also adapt seamlessly to different real-world robots.
RoboCat: The Pioneering AI Model
DeepMind’s research scientist, Alex Lee, explains that RoboCat showcases the unprecedented potential of a single large model. This innovative creation can tackle a wide array of tasks using multiple real robotic embodiments while swiftly adapting to new challenges and environments. In an email interview with TechCrunch, Lee expresses his enthusiasm, stating, “We demonstrate that a single large model can solve a diverse set of tasks on multiple real robotic embodiments and can quickly adapt to new tasks and embodiments.”
Training RoboCat
The development of RoboCat drew inspiration from Gato, another DeepMind AI model renowned for its ability to analyze and respond to text, images, and events. The training process involved leveraging a combination of simulated and real-world data collected from robotics.
To initiate RoboCat’s training, DeepMind researchers collected between 100 to 1,000 demonstrations of a particular task or robot. These demonstrations were conducted using a robotic arm controlled by a human operator. The tasks included activities like picking up gears or stacking blocks. The team then fine-tuned RoboCat on the given task, creating a specialized “spin-off” model. This spin-off model practiced the task an average of 10,000 times, refining its performance.
Expanding RoboCat’s Training Dataset
By amalgamating the data generated from the spin-off models and the initial demonstration data, the researchers continually expanded RoboCat’s training dataset. They trained subsequent versions of RoboCat using this enhanced dataset. Ultimately, the final version of RoboCat was trained on a remarkable total of 253 tasks. To ensure its versatility, RoboCat was benchmarked against a set of 141 variations of these tasks, both in simulation and the real world.
DeepMind claims that after analyzing 1,000 human-controlled demonstrations collected over several hours, RoboCat successfully acquired the capability to operate various types of robotic arms. Despite being trained on robots with two-pronged arms, RoboCat remarkably adapted to a more complex arm featuring a three-fingered gripper and twice as many controllable inputs.
Performance and Future Prospects
DeepMind’s rigorous testing of RoboCat revealed varying success rates across different tasks. Success rates ranged from 13% on the lower end to an impressive 99% on the higher end. These results were obtained with 1,000 demonstrations in the training data, and as expected, success rates were lower when the number of demonstrations was reduced.
However, even with as few as 100 demonstrations, RoboCat displayed the ability to learn new tasks. This suggests that RoboCat has the potential to significantly reduce the barrier to solving new tasks in the field of robotics. Alex Lee shares his optimism about RoboCat’s future, stating, “Provided with a limited number of demonstrations for a new task, RoboCat can be fine-tuned to the new tasks and in turn self-generate more data to improve even further.”
Moving forward, the research team at DeepMind has set an ambitious goal of reducing the number of demonstrations required to teach RoboCat a new task to fewer than 10. This remarkable feat would mark a major breakthrough in robotics and reinforce RoboCat’s position as a trailblazer in the field of adaptive AI.
Conclusion
DeepMind’s RoboCat has revolutionized the world of robotics with its extraordinary ability to tackle diverse tasks and adapt swiftly to new challenges. By combining simulated and real-world data, RoboCat showcases the immense potential of a single large model in robotics. With further advancements and refinement, RoboCat holds the promise of simplifying and expediting the process of teaching robots new tasks. As DeepMind’s groundbreaking creation paves the way for the future of AI-driven robotics, the possibilities are limitless.
Frequently Asked Questions
Q: What is RoboCat?
RoboCat is an AI model developed by DeepMind that possesses the remarkable capability to perform a wide range of tasks using different robotic arms. It is a groundbreaking creation in the field of adaptive AI.
Q: How was RoboCat trained?
DeepMind researchers collected demonstrations of tasks performed by a robotic arm controlled by a human operator. These demonstrations, ranging from 100 to 1,000, were used to fine-tune RoboCat through a specialized “spin-off” model. The final version of RoboCat underwent training on a vast dataset comprising various tasks and benchmarked against numerous task variations.
Q: Can RoboCat adapt to different robotic arms?
Absolutely! Although initially trained on robots with two-pronged arms, RoboCat successfully adapted to a more complex arm featuring a three-fingered gripper and a greater number of controllable inputs.
Q: What success rate does RoboCat achieve across tasks?
The success rate of RoboCat varies across different tasks. DeepMind’s testing revealed success rates ranging from 13% to an impressive 99%. Notably, success rates were influenced by the number of demonstrations available during training.
Q: How will RoboCat impact the field of robotics?
RoboCat has the potential to significantly lower the barrier to solving new tasks in robotics. With its ability to learn new tasks with as few as 100 demonstrations, RoboCat simplifies the process of teaching robots and opens new avenues for advancements in adaptive AI.
Q: What are the future prospects for RoboCat?
DeepMind aims to further enhance RoboCat’s capabilities by reducing the number of demonstrations required to teach it a new task to fewer than 10. This ambitious goal represents a significant stride towards streamlining the integration of AI models in robotics and fostering further innovation.